AACR: Pretrained Machine Learning Models Help Diagnose Nonmelanoma Skin Cancer
By Elana Gotkine HealthDay Reporter
TUESDAY, April 29, 2025 -- Leveraging whole slide embedding from pretrained foundation models (FMs) improves nonmelanoma skin cancer (NMSC) diagnosis, according to a study presented at the annual meeting of the American Association for Cancer Research, held from April 25 to 30 in Chicago.
Spencer Ellis, from the University of Chicago, and colleagues examined the effectiveness of general purpose FMs for diagnosis of NMSC, specifically in resource-constrained environments. Three pathology FMs (UNI, PRISM, and Prov-GigaPath) were evaluated, as was a ResNet18 baseline model. Data were included for 2,130 whole-slide images from 553 suspected NMSC biopsy samples from 455 participants: 706 normal tissue; 638 Bowen disease; 575 basal cell carcinoma; and 211 invasive squamous cell carcinoma.
The researchers found that all three FMs significantly outperformed ResNet18 (mean area under the receiver operating characteristic curve [AUROC], 0.805). The overall best model used the PRISM tile embeddings aggregated using PRISM's intrinsic Perceiver network, which trained a multilayer perceptron (MLP) model to predict the subtype of NMSC (AUROC, 0.925). Using attention-based multi-instance learning to aggregate tile embeddings to train an MLP model was optimal for the UNI and Prov-GigaPath models (mean AUROCs, 0.913 and 0.908, respectively). The simplest method with logistic regression of global average pooling aggregated embeddings could attain reasonable results for PRISM, UNI, and Prov-GigaPath (mean AUROCs, 0.882, 0.865, and 0.855, respectively).
"Our results demonstrate that pretrained machine learning models have the potential to aid diagnosis of NMSC, which might be particularly beneficial in resource-limited settings," coauthor Steven Song, from the Pritzker School of Medicine at the University of Chicago, said in a statement.
Disclaimer: Statistical data in medical articles provide general trends and do not pertain to individuals. Individual factors can vary greatly. Always seek personalized medical advice for individual healthcare decisions.
Source: HealthDay
Posted : 2025-04-30 06:00
Read more
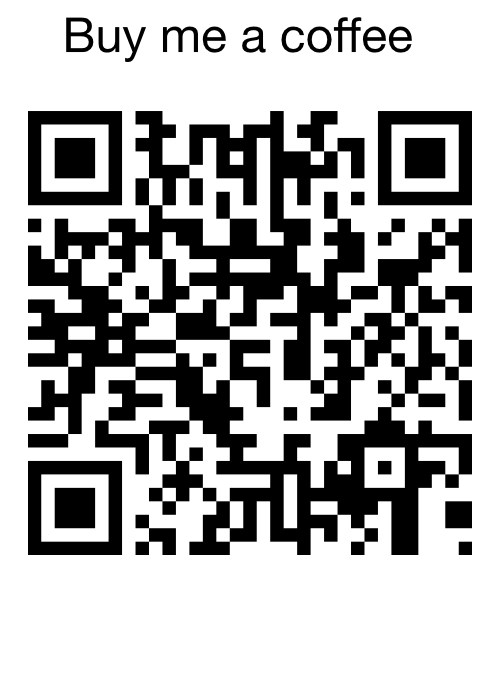
- Infant with Carbamoyl Phosphate Synthetase 1 (CPS1) Deficiency First to Successfully Receive Personalized Gene Therapy Treatment
- 4 States Ask FDA to Lift Rules on Abortion Pill Mifepristone
- Cardiac Deaths Remain Elevated Since 2020
- Less Than Half of Adults Aware of Link Between Alcohol, Cancer
- FDA Approves Nuvaxovid (COVID-19 Vaccine, Adjuvanted) to Prevent COVID-19
- China Donates $500M to WHO as U.S. Pulls Back Support
Disclaimer
Every effort has been made to ensure that the information provided by Drugslib.com is accurate, up-to-date, and complete, but no guarantee is made to that effect. Drug information contained herein may be time sensitive. Drugslib.com information has been compiled for use by healthcare practitioners and consumers in the United States and therefore Drugslib.com does not warrant that uses outside of the United States are appropriate, unless specifically indicated otherwise. Drugslib.com's drug information does not endorse drugs, diagnose patients or recommend therapy. Drugslib.com's drug information is an informational resource designed to assist licensed healthcare practitioners in caring for their patients and/or to serve consumers viewing this service as a supplement to, and not a substitute for, the expertise, skill, knowledge and judgment of healthcare practitioners.
The absence of a warning for a given drug or drug combination in no way should be construed to indicate that the drug or drug combination is safe, effective or appropriate for any given patient. Drugslib.com does not assume any responsibility for any aspect of healthcare administered with the aid of information Drugslib.com provides. The information contained herein is not intended to cover all possible uses, directions, precautions, warnings, drug interactions, allergic reactions, or adverse effects. If you have questions about the drugs you are taking, check with your doctor, nurse or pharmacist.
Popular Keywords
- metformin obat apa
- alahan panjang
- glimepiride obat apa
- takikardia adalah
- erau ernie
- pradiabetes
- besar88
- atrofi adalah
- kutu anjing
- trakeostomi
- mayzent pi
- enbrel auto injector not working
- enbrel interactions
- lenvima life expectancy
- leqvio pi
- what is lenvima
- lenvima pi
- empagliflozin-linagliptin
- encourage foundation for enbrel
- qulipta drug interactions